Tianjian Li
Center for Language and Speech Processing, Johns Hopkins University
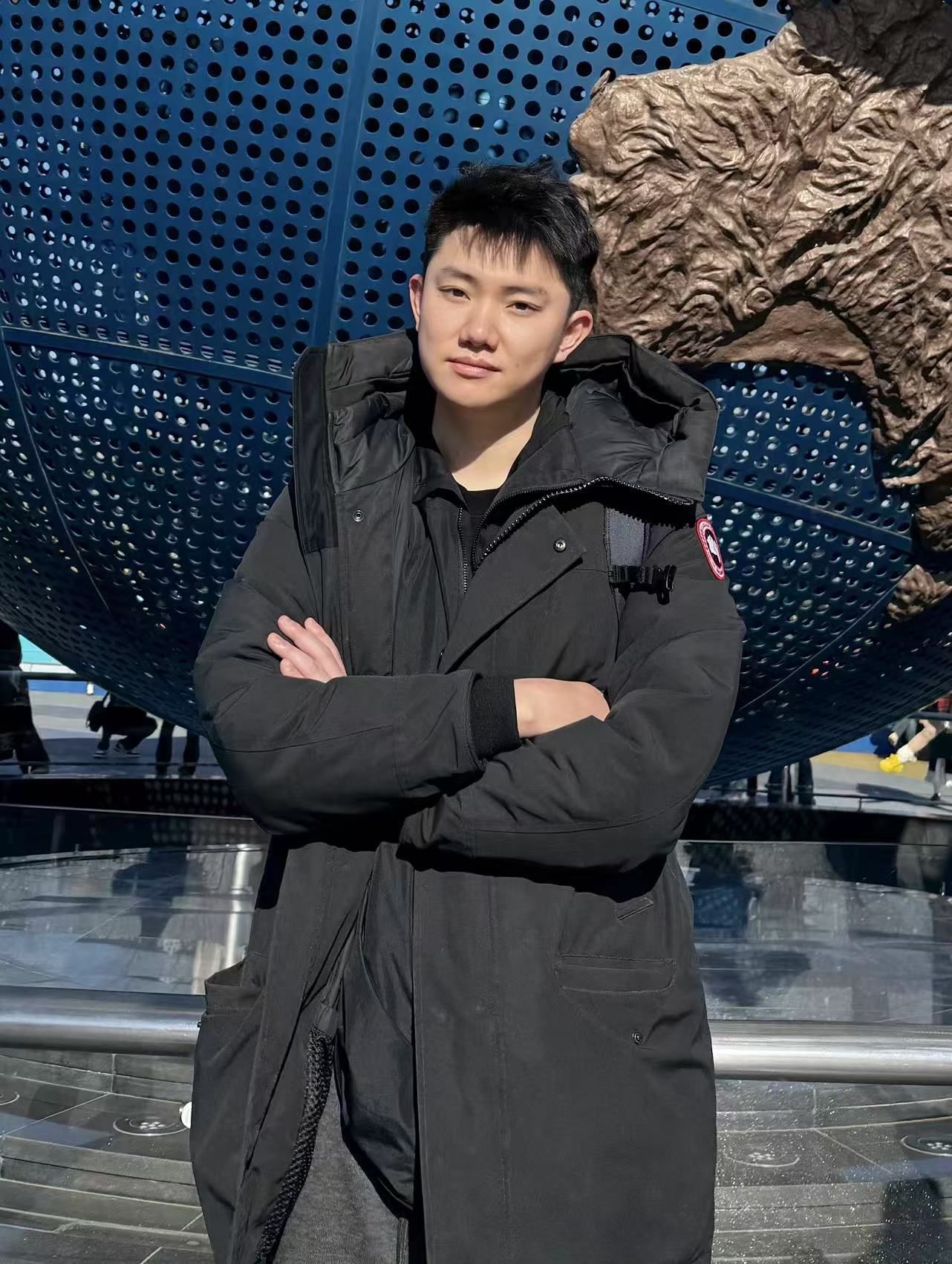
Hi👋, I’m Tianjian! I’m a PhD student in Computer Science at Johns Hopkins University, proudly advised by Prof. Daniel Khashabi. Previously, I completed my Master’s degree in Computer Science at JHU, where I had the privilege of working closely with my wonderful advisors, Kenton Murray and Philipp Koehn. Before that, I was an undergraduate at New York University.
My research lies at the intersection between machine learning and natural language processing.
I prefer solutions that are simple, generalizable, and theoretically sound.
If you have anything to share with me, please feel free to contact me through my email: tli104 at jhu.edu
news
Jan 23, 2025 | 3 papers are accepted to NAACL🎉, which includes my work on training on heavily imbalanced datasets, Jack’s work on making language models produce verbatim quotes from training data, and Yining’s work on evaluating the creativity of language models on code generation. I am super grateful to my wonderful co-authors! |
---|---|
Dec 11, 2024 | I will be joining Meta AI Research (FAIR) as a research intern in summer 2025! |
Dec 6, 2024 | New blog post on why does the chosen and the rejected log-probs is decreased during DPO and why it is to some extent beneficial for alignment. |
Oct 4, 2024 | New preprint on how to train on heavily imbalanced datasets!! |
Apr 7, 2024 | I will be staying at Johns Hopkins University for my PhD, working with Prof. Daniel Khashabi! |
selected publications
- NAACLUpsample or Upweight? Balanced Training on Heavily Imbalanced DatasetsIn Annual Conference of the Nations of the Americas Chapter of the Association for Computational Linguistics (NAACL), 2025
- NAACLBenchmarking Language Model Creativity: A Case Study on Code GenerationIn Annual Conference of the Nations of the Americas Chapter of the Association for Computational Linguistics (NAACL), 2025
- NAACLVerifiable by Design: Aligning Language Models to Quote from Pre-Training DataIn Annual Conference of the Nations of the Americas Chapter of the Association for Computational Linguistics (NAACL), 2025
- ICLRError Norm Truncation: Robust Training in the Presence of Data Noise for Text Generation ModelsIn The Twelfth International Conference on Learning Representations (ICLR)
(Spotlight - Top 5%), 2024 - ACLWhy Does Zero-shot Cross-lingual Generation Fail? An Explaination and A SolutionIn Proceedings of the 2023 Annual Meeting of the Association for Computational Linguistics (ACL Findings), Jul 2023